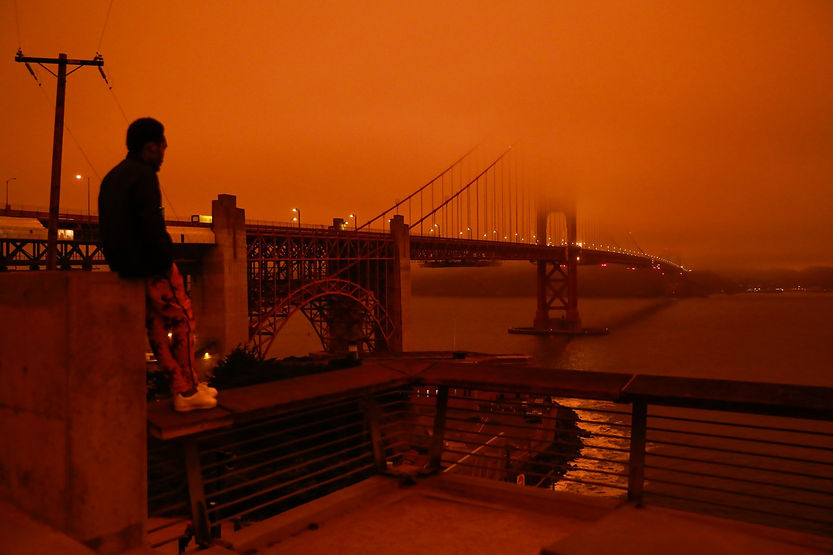
Journey
​
On August 16, 2020, amidst a period of anomalously high temperatures and dry weather, lightning struck the Bay Area. As I stayed up into the early hours of the morning photographing the sparking night sky, the storm moved closer and closer inland. ​
​
Within hours, a lingering metallic smell permeated the air. Within days, the 7000+ wildfires in California had become international news. The SCU Lightning Complex Fire, in particular, would go down as one of the largest fires in California history. Pushing through Alameda County, it was a few miles away from forcing my family to evacuate. By September 9, the blue California sky had turned into a burning and apocalyptic orange, a harrowing reminder of the looming danger of climate change.
​
Wildfires are one of the most preventable natural disasters due to their unique origin. If detected early, firefighters and decision-makers can manage them effectively. However, current methods are not equipped for early, automatic, and continuous wildfire detection.
​
Intrigued by triangulation technology for earthquakes, I played with the idea of applying it to wildfires. What if, instead of seismometers, there were wildfire detectors scattered across a region that could each estimate the wildfire proximity? Then, wildfires could be detected immediately, and their positions could be continuously updated. There was a problem though: there was no such thing as a wildfire detector.
However, thermometers, barometers, anemometers, and dozens of other sensors exist. I had experience conducting research and a passion for machine learning and designing algorithms, and these concepts inspired my hypothesis that deep learning could potentially map meteorological readings to wildfire distance. In other words, I wanted to devise an algorithm that could use measurements from thermometers and other sensors as input and produce a wildfire proximity estimation as output - a wildfire detector.
​
- Rohan